
A large Australian utility company sought LiveEO’s expertise to address their vegetation management challenges, which included monitoring the potential risks posed by vegetation across their expansive network.
A significant portion of this network is situated in remote and sparsely populated areas, making monitoring a daunting task.
Key Takeaways
Vegetation Height Estimation Challenge
A critical aspect of vegetation risk modeling is accurately determining the height of trees relative to infrastructure.
LiveEO achieves this by creating a Canopy Height Model (CHM) using a Digital Surface Model (DSM), which encodes the heights of the earth’s surface and all objects on it, and a Digital Terrain Model (DTM), which encodes the height of the ground.
By subtracting the DSM from the DTM, a CHM is obtained, showing only the vegetation's height.
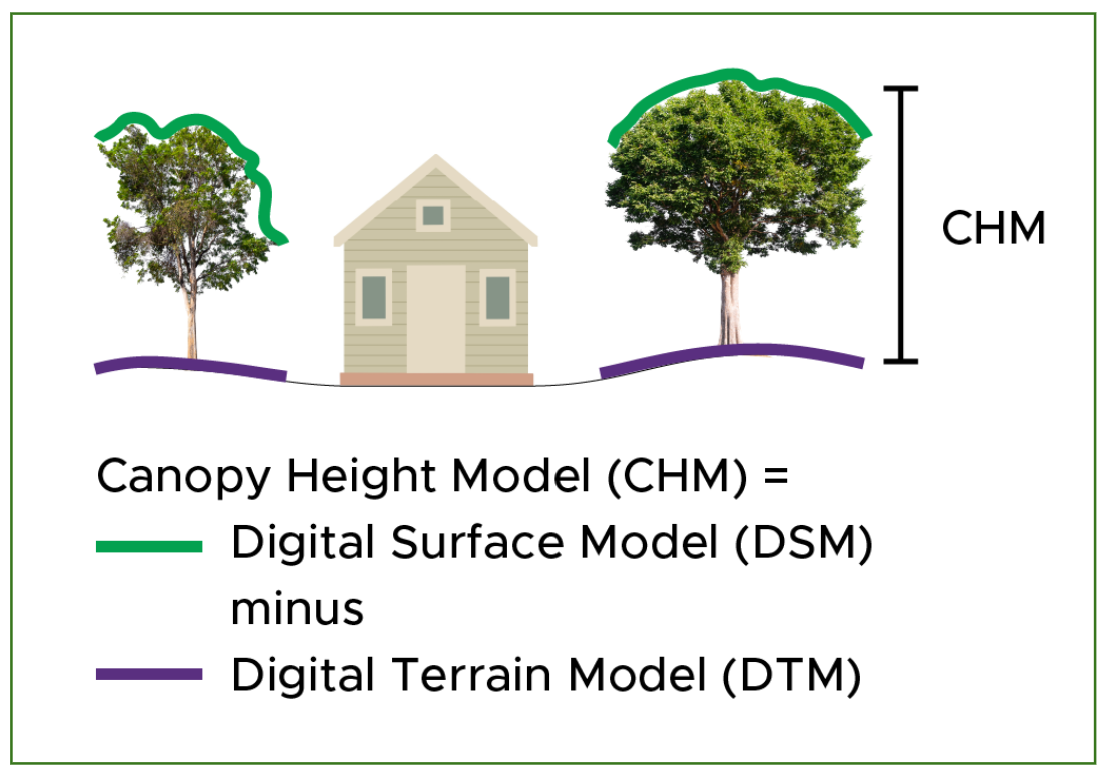
For this project, the DSM was derived from stereoscopic high-resolution satellite imagery and the DTM was derived from customer LiDAR data, with the first iteration using externally-sourced terrain data.
Initially, LiveEO employed traditional photogrammetric methods with classical algorithms for height estimation.
These offered advantages, such as not requiring training for new satellite sensors and being computationally light, leading to faster inference.
Previous tests using these methods in Europe and North America achieved satisfactory results.
However, when LiveEO undertook this project with the Australian client, the limitations of these methods became apparent.
The unique characteristics of Australian vegetation, particularly eucalyptus trees with sparse canopies and smaller leaves, presented difficulties for classical stereo matching algorithms.
As a result, only a mere 5% to 10% of tree canopies could be reconstructed appropriately, rendering them unsuitable for the accurate risk assessment the customer required.
Deep Learning: A Game-Changing Solution
Deep learning has brought about transformative advancements in various fields, such as computer vision, natural language processing, speech recognition, and robotics.
In the context of vegetation management, deep learning enables algorithms to interpret images more like humans do.
It can identify specific features, even when images have varying colors or angles. This capability plays a crucial role in accurately reconstructing 3D structures of vegetation, making it highly effective for monitoring and managing vegetation risks.
Despite its immense potential, deep learning does come with challenges that needed to be addressed.
As the name suggests, deep learning algorithms learn from vast amounts of data and require extensive training to solve complex problems.
Unlike traditional algorithms, deep learning algorithms must be taught, and the training data is essential for their effectiveness. In the case of 3D reconstruction for vegetation management, the highest quality data available comes from aerial LiDAR-derived height models.
LiDAR, which involves data collection through plane, helicopter, or drone flights, enables height estimation within a few tens of centimeters. However, LiDAR collections can be slow, expensive, and challenging to interpret.
To overcome these challenges, LiveEO combined LiDAR data with stereo satellite imagery taken at similar times to provide training data for the deep learning algorithms.
The goal was to achieve the precision of LiDAR while leveraging the cost-effectiveness and scalability of satellite imagery. Creating a substantial database of satellite images as inputs and LiDAR-derived DSMs as targets, LiveEO trained cutting-edge deep learning models to produce higher quality DSMs.
Read also: Combining Satellite Data and LiDAR for UVM (pt 1/3)
Transformative Results
After extensive data collection and model training, the results were remarkable.
The deep learning-based approach vastly outperformed traditional algorithms, accurately reconstructing nearly all trees and closely matching LiDAR-derived heights.
This implies that accurate height estimation can be performed at a fraction of the cost of additional LiDAR overflights.
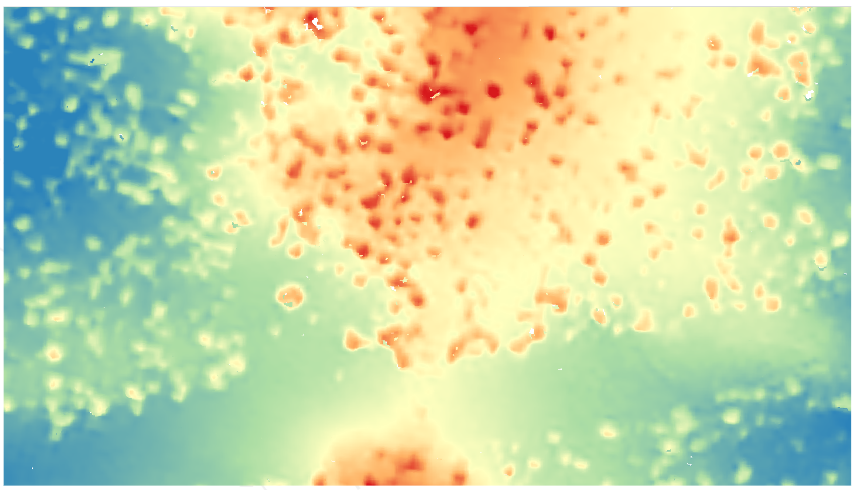
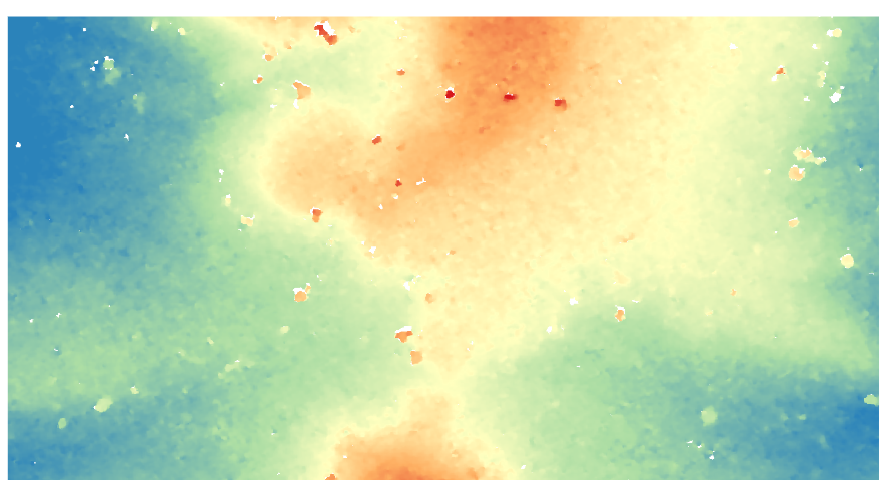
LiveEO's Deep Learning models produced heights closely matching LiDAR-derived heights.

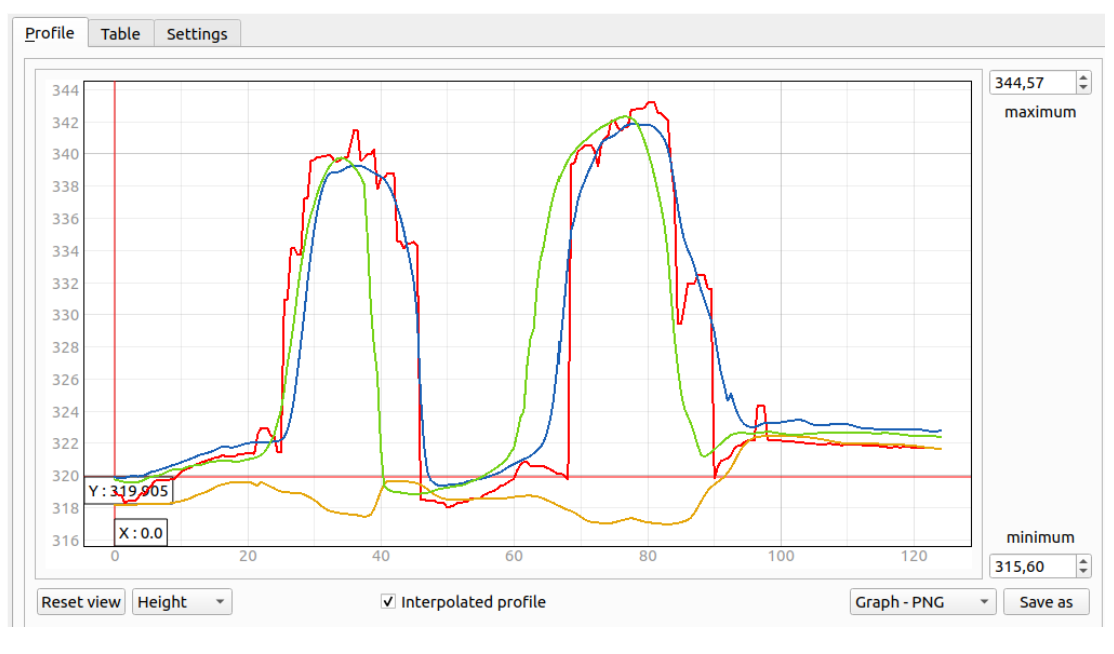
Blue: DL - PNeo
Green: DL - Pleiades
Yellow: Traditional photogrammetry (Pleiades)
This groundbreaking technology empowers LiveEO to create precise vegetation height models globally, regardless of location or scale.
With deep learning's ability to understand images in a more meaningful way, LiveEO now offers an even more powerful solution for vegetation risk assessment, enhancing safety and reliability across the utility company's infrastructure network, as well as enabling companies to get the most out of their existing LiDAR data.
Talk to one of our experts and explore the potential of deep learning in enhancing your vegetation risk assessment.